Raymond Sol; Carlin C.F. Chu; Jessie C.W. Lee
The emergence of transformer-based pre-trained language models (PTLMs) has brought new and improved techniques to natural language processing (NLP). Traditional rule-based NLP, for instance, is known for its deficiency of creating context-aware representations of words and sentences. Natural language inference (NLI) addresses this deficiency by using PTLMs to create context-sensitive embedding for contextual reasoning. This paper outlines a system design that uses traditional rule-based NLP and deep learning to extract aspect-based financial opinion from financial commentaries written using colloquial Cantonese, a dialect of the Chinese language used in Hong Kong. We need to confront the issue that existing off-the-shelf PTLMs, such as BERT and Roberta, are not pre-trained to understand the language semantics of colloquial Cantonese, let alone the slang, jargon, and codeword that people in Hong Kong use to articulate opinions. As a result, we approached the opinion extraction problem differently from the mainstream approaches, which use model-based named entity recognition (NER) to detect and extract opinion aspects as named entities and named entity relations.
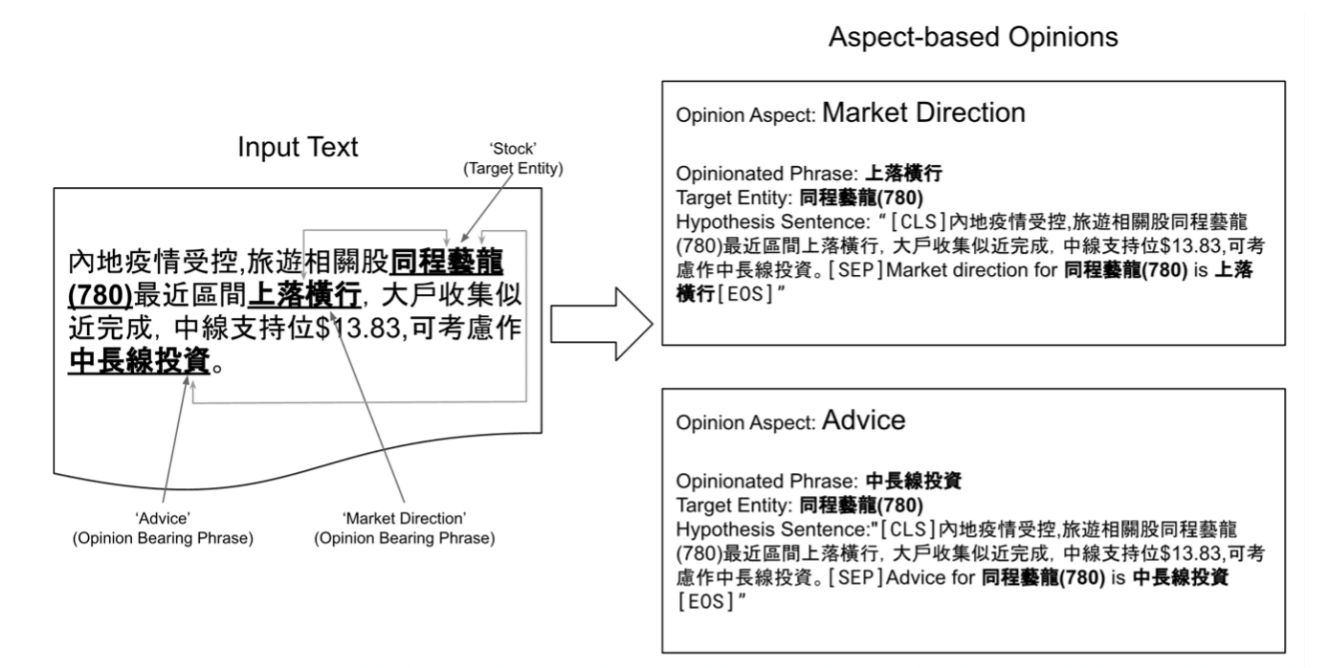